Embodied Information
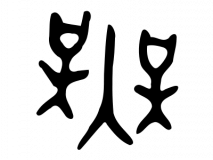
In this project we examine the question of whether any algorithmical resp. non-algorithmical concept as well as any kind of scientific theory are ever be incomplete. We investigate this question with particular attention to the claims made that these concepts can contribute to the notions of observation, prediction, recollection and explanation. There’s some evidence about an interrelation between ideas within the philosophy of science – the Duhem-Quine thesis of underdetermination of observations and the observational/theoretical terms distinction failure – and the well known limitative theorems of Gödel and Tarski etc. Although these results original apply to deduction from axioms, we have a further evidence to assume that their extensions hold for general inference devices, i.e. recursive enumerable ones (like structural inductive) as well, as for other possible systems of logic with non-recursive sets of axioms resp. rules of inference and furthermore for any constraint satisfaction problem. This would imply that any idea or concept and any experience cannot be completely defined or contextualized. – So there’s a strong sense in which we remain under the shadow of chance and randomness.
The aim of this research work is to review, clarify, and critically analyze aspects of modern mathematical information theories. The emphasis is upon mathematical structures involved, rather than numerical computations. We will argue that theories and concepts of information and complexity can never be complete. For that reason particular attention will be paid to various provided measures of information and complexity and their dependence on algorithmical, resp. non-algorithmical concepts. We try to reveal the supposed conditions for incompleteness like computational irreducibility, arbitrariness, infinity, and self-awareness. Working hypothesis is that due to connections with disguised forms of the meta-mathematical theorems of Gödel and Tarski incompleteness is widely an epistemological limit which is manifest, e.g. in the non-existence of a procedure to determine valid empirical observations resp. the undefinability of valid observations, and we assume that this limit is not likely to be broken any time soon.
Website
http://www.geisteswissenschaften.fu-berlin.de/v/embodiedinformation/
Contact
Prof. Dr. Georg Trogemann
Professor for Experimental Computer Science
Phone: +49 – (0)221 – 20189 – 131
Fax: +49 – (0)221 – 20189 – 230
Mail: trogemann@khm.de
Picture Credits
http://commons.wikimedia.org/wiki/Media:?-oracle.svg
Image depicting the character Mu in the ancient chinese oracle script. Mu or wú is a word which has been roughly translated as “no”, “none”, “null”, “without”, “no meaning”. In Japanese and Chinese mainly used as a prefix to imply the absence of something. It’s a word meaning neither yes nor no, i.e. this question has no unambiguous answer. Perhaps in the end this idea should be the only answer to the illusory question of lawful explanations of natural phenomena?
References
(Keywords: Computation and Information, Entropie and Information, Physics and Information, Information Geometry, Information Theory, Algorithmic Information, Algorithmic Probability, Algorithmic Randomness, Complexity, Probability Theory, Probability and Measure, Statistical Inference, Induction)
- Radoslaw Adamczak, “A tail inequality for suprema of unbounded empirical processes with applications to Markov chains”, arxiv:0709.3110
- David Z. Albert, Time and Chance, Harvard UP, 2000
- Sergio Albeverio, Song Liang, “Asymptotic expansions for the Laplace approximations of sums of Banach space-valued random variables”, Annals of Probability 33 (2005): 300–336 = math.PR/0503601
- David J. Aldous, Antar Bandyopadhyay, “A survey of max-type recursive distributional equations”, math.PR/0401388 = Annals of Applied Probability 15 (2005): 1047–1110
- Paul H. Algoet, Thomas M. Cover, “A Sandwich Proof of the Shannon-McMillan-Breiman Theorem”, Annals of Probability 16 (1988): 899–909 [a asymptotic equipartition result for relative entropy …]
- Robert Alicki, “Information-theoretical meaning of quantum dynamical entropy,” quant-ph/0201012
- Armen E. Allahverdyan, “Entropy of Hidden Markov Processes via Cycle Expansion”, arxiv:0810.4341
- A. E. Allahverdyan, Th. M. Nieuwenhuizen, “Breakdown of the Landauer bound for information erasure in the quantum regime,” cond-mat/0012284
- P. Allegrini, V. Benci, P. Grigolini, P. Hamilton, M. Ignaccolo, G. Menconi, L. Palatella, G. Raffaelli, N. Scafetta, M. Virgilio, J. Jang, “Compression and diffusion: a joint approach to detect complexity,” cond-mat/0202123
- Sun-Ichi Amari, “Information Geometry on Hierarchy of Probability Distributions”, IEEE Transacttions on Information Theory 47 (2001): 1701–1711 [PDF reprint]
- Sun-Ichi Amari, Hiroshi Nagaoka, Methods of Information Geometry, (2001),Vol. 191. AMS
- Jose M. Amigo, Matthew B. Kennel, Ljupco Kocarev, “The permutation entropy rate equals the metric entropy rate for ergodic information sources and ergodic dynamical systems”, nlin.CD/0503044
- David Applebaum, Probability and Information: An Integrated Approach, Cambridge UP, 1996
- Khadiga Arwini, C. T. J. Dodson, “Neighborhoods of Independence for Random Processes”, math.DG/0311087
- Nihat Ay
“Information geometry on complexity and stochastic interaction” [preprint]
“An information-geometric approach to a theory of pragmatic structuring” [preprint]
- Massimiliano Badino, “An Application of Information Theory to the Problem of the Scientific Experiment”, Synthese 140 (2004): 355–389 [but specifies the theory completely the probability of observations, i.e., no free parameters to be estimated from data? …whats going on with any other of the work relating information theory to hypothesis testing at least from Kullback in the 1950s… MS Word preprint]
- Vijay Balasubramanian, “Statistical Inference, Occam’s Razor, and Statistical Mechanics on the Space of Probability Distributions”, Neural Computation 9 (1997): 349–368
- David Balding, Pablo A. Ferrari, Ricardo Fraiman, Mariela Sued, “Limit theorems for sequences of random trees”, math.PR/0406280 [Abstract: ” We consider a random tree and introduce a metric in the space of trees to define the “mean tree” as the tree minimizing the average distance to the random tree. When the resulting metric space is compact we show laws of large numbers and central limit theorems for sequence of independent identically distributed random trees. As application we propose tests to check if two samples of random trees have the same law.”]
- R. Balian, “Information in statistical physics”, cond-mat/0501322
- K. Bandyopadhyay, A. K. Bhattacharya, Parthapratim Biswas, D. A. Drabold, “Maximum entropy and the problem of moments: A stable algorithm”, cond-mat/0412717
- Richard G. Baraniuk, Patrick Flandrin, Augustus J. E. M. Janssen, Olivier J. J. Michel, “Measuring Time-Frequency Information Content Using the Renyi Entropies”, IEEE Transactions on Information Theory 47 (2001): 1391–1409
- M. Maissam Barkeshli, “Dissipationless Information Erasure and the Breakdown of Landauer’s Principle”, cond-mat/0504323
- O. E. Barndorff-Nielsen, Richard D. Gill, “Fisher Information in Quantum Statistics”, quant-ph/9808009
- Andrea Baronchelli, Emanuele Caglioti, Vittorio Loreto, “Artificial sequences and complexity measures”, Journal of Statistical Mechanics: Theory and Experiment (2005): P04002
- John Bates, Harvey Shepard, “Measuring Complexity Using Information Fluctuation,” Physics Letters A 172 416–425 (1993)
- Felix Belzunce, Jorge Navarro, José M. Ruiz, Yolanda del Aguila, “Some results on residual entropy function”, Metrika 59 (2004): 147–161
- Fabio Benatti, Tyll Krueger, Markus Mueller, Rainer Siegmund-Schultze, Arleta Szkola, “Entropy and Algorithmic Complexity in Quantum Information Theory: a Quantum Brudno’s Theorem”, quant-ph/0506080
- Charles H. Bennett “Dissipation, Information, Computational Complexity and the Definition of Organization,” in David Pines (ed.), Emerging Syntheses in Science, Westview Press, 1988
- Charles H. Bennett “How to Define Complexity in Physics, and Why” in W. H. Zurek (ed.), Complexity, Entropy, and the Physics of Information (1991) Proceedings of the 1988 Workshop on Complexity, Entropy, and the Physics of Information, held May-June, 1989 in Santa Fe, New Mexico
- Charles H. Bennett, “Notes on Landauer’s principle, reversible computation, and Maxwell’s Demon”, Studies In History and Philosophy of Science Part B 34 (2003): 501–510
- Carl T. Bergstrom, Michael Lachmann, “The fitness value of information”, q-bio.PE/0510007
- Patrick Billingsley, Probability and Measure, Wiley & Sons, N.Y.C. 1995
- Igor Bjelakovic, Tyll Krueger, Rainer Siegmund-Schultze, Arleta Szkola
“The Shannon-McMillan Theorem for Ergodic Quantum Lattice Systems,” math.DS/0207121
“Chained Typical Subspaces – a Quantum Version of Breiman’s Theorem,” quant-ph/0301177
- Gunnar Blom, Lars Holst, Dennis Sandell, Problems and Snapshots from the World of Probability, N.Y.C., Springer 1994
- Claudio Bonanno, “The Manneville map: topological, metric and algorithmic entropy,” math.DS/0107195
- Brillouin, Science and Information Theory, Dover Phoenix Editions: Dover Publications, 1962
- Paul Bohan Broderick, “On Communication and Computation”, Minds and Machines 14 (2004): 1–19 [“The most famous models of computation and communication, Turing Machines and (Shannon-style) information sources, are considered. The most significant difference lies in the types of state-transitions allowed in each sort of model. This difference does not correspond to the difference that would be expected after considering the ordinary usage of these terms.”]
- Kenneth P. Burnham, David R. Anderson, Model Selection and Inference: A Practical Information-Theoretic Approach
- Xavier Calmet, Jacques Calmet, “Dynamics of the Fisher Information Metric”, cond-mat/0410452 = Physical Review E 71 (2005): 056109
- C. J. Cellucci, A. M. Albano, P. E. Rapp, “Statistical validation of mutual information calculations: Comparison of alternative numerical algorithms”, Physical Review E 71 (2005): 066208
- Massimo Cencini, Alessandro Torcini, “A nonlinear marginal stability criterion for information propagation,” nlin.CD/0011044
- Gregory J. Chaitin
Algorithmic Information Theory [online]
Information, Randomness and Incompleteness [online]
Information-Theoretic Incompleteness [online]
- Sourav Chatterjee, “A new method of normal approximation”, arxiv:math/0611213
- Bernard Chazelle, The Discrepency Method: Randomness and Complexity, Cambridge UP, 2000
- J.-R. Chazottes, D. Gabrielli, “Large deviations for empirical entropies of Gibbsian sources”, math.PR/0406083 = Nonlinearity 18 (2005): 2545–2563 [large deviation principle in block entropies, and entropy rates estimated from those blocks – even as one lets the length of the blocks grow with the amount of data, provided the block-length doesn’t grow too quickly (only ln_2)]
- J.-R. Chazottes, E. Uglade, “Entropy estimation and fluctuations of Hitting and Recurrence Times for Gibbsian sources”, math.DS/0401093
- Tommy W. S. Chow, D. Huang, “Estimating Optimal Feature Subsets Using Efficient Estimation of High-Dimensional Mutual Information”, IEEE Transactions on Neural Networks 16 (2005): 213–224
- Bob Coecke, “Entropic Geometry from Logic,” quant-ph/0212065
- Thomas M. Cover, Joy A. Thomas, Elements of Information Theory, Wiley Series in Telecommunications and Signal Processing, 2006
- Gavin E. Crooks, “Measuring Thermodynamic Length”, Physical Review Letters 99 (2007): 100602 [“Thermodynamic length is a metric distance between equilibrium thermodynamic states. Among other interesting properties, this metric asymptotically bounds the dissipation induced by a finite time transformation of a thermodynamic system. It is also connected to the Jensen-Shannon divergence, Fisher information, and Rao’s entropy differential metric.”]
- Alfred Crosby, The Measure of Reality, Cambridge University Press, 1997
- Imre Csiszar, “The Method of Types”, IEEE Tranactions on Information Theory<.cite> 44 (1998): 2505–2523 [PDF]
- Imre Csiszar, Janos Korner, Information Theory: Coding Theorems for Discrete Memoryless Systems, Academic Press 1997
- Imre Csiszar, Frantisek Matus, “Closures of exponential families”, Annals of Probability 33 (2005): 582–600 = math.PR/0503653
- Imre Csiszar, Paul Shields, Information Theory and Statistics: A Tutorial [Fulltext PDF]
- A. Daffertshofer, A. R. Plastino, “Landauer’s principle and the conservation of information”, Physics Letters A 342 (2005): 213–216
- Lukasz Debowski, “On vocabulary size of grammar-based codes”, cs.IT/0701047
- Gustavo Deco, Bernd Schurmann, Information Dynamics: Foundations and Applications, Springer, Berlin 2000
- Morris DeGroot, Mark J. Schervish, Probability and Statistics, Addison Wesley, 2001
- F. M. Dekking, C. Kraaikamp, H. P. Lopuhaä, L. E. Meester, A Modern Introduction to Probability and Statistics: Understanding How and Why [>>>]
- Victor De La Pena, Evarist Gine, Decoupling: From Dependence to Independence, Springer, 1998
- Victor H. de la Pena, Tze Leung Lai, Qi-Man Shan, Self-Normalized Processes: Limit Theory and Statistical Applications [>>>]
- Amir Dembo, “Information Inequalities and Concentration of Measure”, The Annals of Probability 25 (1997): 927–939 [“We derive inequalities of the form \Delta(P,Q) =< H(P|R) + H(Q|R) which hold for every choice of probability measures P, Q, R, where H(P|R) denotes the relative entropy of P with respect to R and \Delta(P,Q) stands for a coupling type ‘distance’ between P and Q.”]
- Amir Dembo, I. Kontoyiannis, “Source Coding, Large Deviations, and Approximate Pattern Matching,” math.PR/0103007
- Steffen Dereich, “The quantization complexity of diffusion processes”, math.PR/0411597
- Joseph DeStefano, Erik Learned-Miller, “A Probabilistic Upper Bound on Differential Entropy”, cs.IT/0504091 [“A novel, non-trivial, probabilistic upper bound on the entropy of an unknown one-dimensional distribution, given the support of the distribution and a sample from that distribution…”]
- Persi Diaconis, Svante Janson, “Graph limits and exchangeable random graphs”, arxiv:0712.2749
- David Doty, “Every sequence is compressible to a random one”, cs.IT/0511074 [“Kucera and Gacs independently showed that every infinite sequence is Turing reducible to a Martin-Löf random sequence. We extend this result to show that every infinite sequence S is Turing reducible to a Martin-Löf random sequence R such that the asymptotic number of bits of R needed to compute n bits of S, divided by n, is precisely the constructive dimension of S.”]
- David Doty, Jared Nichols, “Pushdown Dimension”, cs.IT/0504047
- D. Dowe, K. Korb, J. Oliver (eds.), Information, Statistics and Induction in Science, Singapore: World Scientific Publishing Company, 1996
- Alvin W. Drake, Fundamentals of Applied Probability Theory, Mcgraw-Hill College 1967
- M. Drmota, W. Szpankowski, “Precise minimax redundancy and regret”, IEEE Transactions on Information Theory 50 (2004): 2686–2707
- Ray Eames, Charles Eames, A Communications Primer [short film 1953]
- John Earman, John Norton, “Exorcist XIV: The wrath of Maxwell’s Demon”
“From Maxwell to Szilard”, Studies in the History and Philosophy of Modern Physics 29 (1998): 435–471
“From Szilard to Landauer and beyond”, Studies in the History and Philosophy of Modern Physics 30 (1999): 1–40
- Bruce R. Ebanks, Prasanna Sahoo, Wolfgang Sander, Characterization of Information Measures, Springer, 1998
- Karl-Erik Eriksson, Kristian Lindgren, Bengt Å. Månsson, Structure, Context, Complexity, Organization: Physical Aspects of Information and Value, Singapore : World Scientific, 1987
- Dave Feldman, Information Theory, Excess Entropy and Statistical Complexity
- William Feller, An Introduction to Probability Theory and Its Applications, Wiley, 1968
- J. M. Finn, J. D. Goettee, Z. Toroczkai, M. Anghel, B. P. Wood, “Estimation of entropies and dimensions by nonlinear symbolic time series analysis”, Chaos 13 (2003): 444–456
- John W. Fisher III, Alexander T. Ihler, Paula A. Viola, “Learning Informative Statistics: A Nonparametric Approach”, pp. 900–906 in NIPS 12 (1999) [PDF reprint]
- P. Flocchini et al. (eds.), Structure, Information and Communication Complexity, Proceedings of 1st International Conference on Structural Information and Communication Complexity, Ottawa, May 1994, Carleton UP, 1995.
- Bert Fristedt, Lawrence Gray, A Modern Approach to Probability Theory, Birkhäuser, Boston, 1997
- Peter Gács, “Uniform test of algorithmic randomness over a general space”, Theoretical Computer Science 341 (2005): 91–137 [“The algorithmic theory of randomness is well developed when the underlying space is the set of finite or infinite sequences and the underlying probability distribution is the uniform distribution or a computable distribution. These restrictions seem artificial. Some progress has been made to extend the theory to arbitrary Bernoulli distributions (by Martin-Löf) and to arbitrary distributions (by Levin). We recall the main ideas and problems of Levin’s theory, and report further progress in the same framework….”]
- Travis Gagie, “Compressing Probability Distributions”, cs.IT/0506016 [Abstract (in full): “We show how to store good approximations of probability distributions in small space.”]
- Janos Galambos, Italo Simonelli, Bonferroni-type Inequalities with Applications, Springer, Berlin, 1996
- Stefano Galatolo, Mathieu Hoyrup, Cristóbal Rojas, “Effective symbolic dynamics, random points, statistical behavior, complexity and entropy”, arxiv:0801.0209 [All, not almost all, Martin-Löf points are statistically typical.]
- Yun Gao, Ioannis Kontoyiannis, Elie Bienenstock, “From the entropy to the statistical structure of spike trains”, arxiv:0710.4117
- Pierre Gaspard, “Time-Reversed Dynamical Entropy and Irreversibility in Markovian Random Processes”, Journal of Statistical Physics 117 (2004): 599–615
- David Gelernter, Mirror Worlds, Oxford UP, 1993
- George M. Gemelos, Tsachy Weissman, “On the Entropy Rate of Pattern Processes”, cs.IT/0504046
- Neil Gershenfeld, The Physics of Information Technology, Cambridge UP, 2000
- Paolo Gibilisco, Tommaso Isola, “Uncertainty Principle and Quantum Fisher Information”, math-ph/0509046
- Paolo Gibilisco, Daniele Imparato, Tommaso Isola, “Uncertainty Principle and Quantum Fisher Information II” math-ph/0701062
- Josep Ginebra, “On the Measure of the Information in a Statistical Experiment”, Bayesian Analysis (2007): 167–212
- Clark Glymour, “Instrumental Probability”, Monist 84 (2001): 284–300 [PDF reprint]
- M. Godavarti, A. Hero, “Convergence of Differential Entropies”, IEEE Transactions on Information Theory 50 (2004): 171–176
- Stanford Goldman, Information Theory, Dover Publ., 1969 [some interesting time-series which has dropped out of most modern presentations]
- J. A. Gonzalez, L. I. Reyes, J. J. Suarez, L. E. Guerrero, G. Gutierrez, “A mechanism for randomness,” nlin.CD/0202022
- H. Gopalkrishna Gadiyar, K. M. Sangeeta Maini, R. Padma, H. S. Sharatchandra, “Entropy and Hadamard matrices”, Journal of Physics A: Mathematical and General 36 (2003): L109–L112
- Alexander N. Gorban, Iliya V. Karlin, Hans Christian Ottinger, “The additive generalization of the Boltzmann entropy,” cond-mat/0209319 [a rediscovery of Renyi entropies…?]
- Peter Grassberger “Randomness, Information, and Complexity,” pp. 59–99 of Francisco Ramos-Gómez (ed.), Proceedings of the Fifth Mexican School on Statistical Physics (Singapore: World Scientific, 1989)
- Peter Grassberger, “Data Compression and Entropy Estimates by Non-sequential Recursive Pair Substitution,” physics/0207023
- R. M. Gray, Entropy and Information Theory [on-line.]
- Andreas Greven, Gerhard Keller, Gerald Warnecke (eds.), Entropy, Princeton UP, 2003
- Geoffrey R. Grimmett, David Stirzaker, Probability and Random Processes, Oxford UP, 2001
- Peter Grünwald, Paul Vitányi, “Shannon Information and Kolmogorov Complexity”, cs.IT/0410002
- Sudipto Guha, Andrew McGregor, Suresh Venkatasubramanian, “Streaming and Sublinear Approximation of Entropy and Information Distances”, 17th ACM-SIAM Symposium on Discrete Algorithms, 2006 [Link via Suresh]
- Ian Hacking
The Emergence of Probability, Cambridge UP, 2006
The Taming of Chance, Cambridge UP, 1990
- Michael J. W. Hall, “Universal Geometric Approach to Uncertainity, Entropy and Information,” physics/9903045
- Guangyue Han, Brian Marcus, “Analyticity of Entropy Rate in Families of Hidden Markov Chains”, math.PR/0507235
- Te Sun Han
“Hypothesis Testing with the General Source”, IEEE Transactions on Information Theory 46 (2000): 2415–2427 = math.PR/0004121 [“The asymptotically optimal hypothesis testing problem with the general sources as the null and alternative hypotheses is studied…. Our fundamental philosophy in doing so is first to convert all of the hypothesis testing problems completely to the pertinent computation problems in the large deviation-probability theory. … [This] enables us to establish quite compact general formulas of the optimal exponents of the second kind of error and correct testing probabbilities for the general sources including all nonstationary and/or nonergodic sources with arbitrary abstract alphabet (countable or uncountable). Such general formulas are presented from the information-spectrum point of view.”]
“Folklore in Source Coding: Information-Spectrum Approach”, IEEE Transactions on Information Theory 51 (2005): 747–753 [From the abstract: “we verify the validity of the folklore that the output from any source encoder working at the optimal coding rate with asymptotically vanishing probability of error looks like almost completely random.”]
“An information-spectrum approach to large deviation theorems”, cs.IT/0606104
- Te Sun Han, Kingo Kobayashi, Mathematics of Information and Coding [>>>]
- Masahito Hayashi, “Second order asymptotics in fixed-length source coding and intrinsic randomness”, cs.IT/0503089
- Nicolai T. A. Haydn, “The Central Limit Theorem for uniformly strong mixing measures”, arxiv:0903.1325
- Nicolai Haydn and Sandro Vaienti, “Fluctuations of the Metric Entropy for Mixing Measures”, Stochastics and Dynamics 4 (2004): 595–627
- Torbjorn Helvik, Kristian Lindgren, Mats G. Nordahl, “Continity of Information Transport in Surjective Cellular Automata”, Communications in Mathematical Physics 272 (2007): 53-74
- Alexander E. Holroyd, Terry Soo, “A Non-Measurable Set from Coin-Flips”, math.PR/0610705
- Hong-Da Chen, Chang-Heng Chang, Li-Ching Hsieh, Hoong-Chien Lee, “Divergence and Shannon Information in Genomes”, Physical Review Letters 94 (2005): 178103
- M. Hotta and I. Jochi, “Composability and Generalized Entropy,” cond-mat/9906377
- Marcus Hutter, “Distribution of Mutual Information,” cs.AI/0112019
Universal artificial intelligence: Sequential Decisions based on algorithmic probability. Springer, Berlin, 2004.
- Marcus Hutter, Marco Zaffalon, “Distribution of mutual information from complete and incomplete data”, Computational Statistics and Data Analysis 48 (2004): 633–657
- Shunsuke Ihara, Information Theory for Continuous Systems, World Scientific Publ., 1993
- Shiro Ikeda, Toshiyuki Tanaka, Shun-ichi Amari, “Stochastic Reasoning, Free Energy, and Information Geometry”, Neural Computation 16 (2004): 1779–1810
- K. Iriyama, “Error Exponents for Hypothesis Testing of the General Source”, IEEE Transactions on Information Theory 51 (2005): 1517–1522
- K. Iwata, K. Ikeada, H. Sakai, “A Statistical Property of Multiagent Learning Based on Markov Decision Process”, IEEE Transactions on Neural Networks 17 (2006): 829–842 [The property is asymptotic equipartiton!]
- Aleks Jakulin, Ivan Bratko, “Quantifying and Visualizing Attribute Interactions”, cs.AI/0308002
- W. Janke, D.A. Johnston, R. Kenna, “Information Geometry and Phase Transitions”, cond-mat/0401092 = Physica A 336 (2004): 181–186
- Petr Jizba, Toshihico Arimitsu, “The world according to Renyi: Thermodynamics of multifractal systems,” cond-mat/0207707
- Oliver Johnson
“A conditional Entropy Power Inequality for dependent variables,” math.PR/0111021
“Entropy and a generalisation of `Poincare’s Observation’,” math.PR/0201273
- Oliver Johnson, Richard Samworth, “Central Limit Theorem and convergence to stable laws in Mallows distance”, math.PR/0406218
- Oliver Johnson, Andrew Barron, “Fisher Information inequalities and the Central Limit Theorem,” math.PR/0111020
- Mark Kac
Engimas of Chance, University of California Press, 1987
Probability and Related Topics in Physical Science, American Mathematical Society, 1957
Statistical Independence in Probability, Analysis and Number Theory, Mathematical Assn of Amer.,1959, Carus Mathematical Monographs, Nr.12
- Olav Kallenberg, Foundations of Modern Probability, Springer, 2002
- Olav Kallenberg, Probabilitic Symmetries and Invariance Principles [“This is the first comprehensive treatment of the three basic symmetries of probability theory – contractability, exchangeability, and rotatability – defined as invariance in distribution under contractions, permutations, and rotations.” >>>]
- Alexei Kaltchenko, “Algorithms for Estimating Information Distance with Applications to Bioinformatics and Linguistics”, cs.CC/0404039
- Robert E. Kass, Paul W. Vos, Geometrical Foundations of Asymptotic Inference, Wiley-Interscience, 1997
- Ido Kanter, Hanan Rosemarin, “Communication near the channel capacity with an absence of compression: Statistical Mechanical Approach,” cond-mat/0301005
- Hillol Kargupta, “Information Transmission in Genetic Algorithm and Shannon’s Second Theorem”, Proceedings of the 5th International Conference on Genetic Algorithms, 1993
- Matthew B. Kennel, Jonathon Shlens, Henry D. I. Abarbanel, E. J. Chichilnisky, “Estimating Entropy Rates with Bayesian Confidence Intervals”, Neural Computation 17 (2005): 1531–1576
- D. F. Kerridge, “Inaccuracy and Inference”, Journal of the Royal Statistical Society B 23 (1961): 184–194
- Shiraj Khan, Sharba Bandyopadhyay, Auroop R. Ganguly, Sunil Saigal, David J. Erickson, Vladimir Protopopescu, George Ostrouchov, “Relative performance of mutual information estimation methods for quantifying the dependence among short and noisy data”, Physical Review E 76 (2007): 026209
- A. I. Khinchin, Mathematical Foundations of Information Theory, Dover Publ., 1957 [an axiomatic approach]
- Andrei Kolmogorov, Foundations of Probability Theory, Chelsea Publ.Co., N.Y.C., 1956
- Alexander Kraskov, Harald Stögbauer, Peter Grassberger, “Estimating Mutual Information”, cond-mat/0305641 = Physical Review E 69 (2004): 066138
- Rudolf Kulhavý Recursive Nonlinear Estimation: A Geometric Approach, (Lecture Notes in Control and Information Sciences. 216). Springer, London 1996
- Solomon Kullback, Information Theory and Statistics, Dover Publ., 1997
- Rolf Landauer, “The Physical Nature of Information,” Physics Letters A 217 (1996): 188–193
- Bernard H. Lavenda, “Information and coding discrimination of pseudo-additive entropies (PAE)”, cond-mat/0403591
- G. Lebanon, “Axiomatic Geometry of Conditional Models”, IEEE Transactions on Information Theory 51 (2005): 1283–1294
- Tue Lehn-schioler, Anant Hegde, Deniz Erdogmus, Jose C. Principe, “Vector quantization using information theoretic concepts”, Natural Computation 4 (2005): 39–51 [“it becomes clear that minimizing the free energy of the system is in fact equivalent to minimizing a divergence measure between the distribution of the data and the distribution of the processing elements, hence, the algorithm can be seen as a density matching method.”]
- Emmanuel Lesigne, Heads or Tails: An Introduction to Limit Theorems in Probability [>>>]
- Christophe Letellier, “Estimating the Shannon Entropy: Recurrence Plots versus Symbolic Dynamics”, Physical Review Letters 96 (2006): 254102
- Lev B. Levitin, “Energy Cost of Information Transmission (Along the Path to Understanding),” Physica D 120(1998): 162–167
- Lev B. Levitin, Tommaso Toffoli, “Thermodynamic Cost of Reversible Computing”, Physical Review Letters 99 (2007): 110502
- M. Li, P. M B. Vitanyi. An introduction to Kolmogorov complexity and its applications. Springer, N.Y.C.,1997(2007).[>>>]
- F. Liang, A. Barron, “Exact Minimax Strategies for Predictive Density Estimation, Data Compression, and Model Selection”, IEEE Transactions on Information Theory 50 (2004): 2708–2726
- Douglas Lind, Brian Marcus, Symbolic Dynamics and Coding, Cambridge UP, 1995
- Christian Lindgren, “Information Theory for Complex Systems” (Online lecture notes, dated Jan. 2003)
- Seth Lloyd
“Use of Mutual Information to Decrease Entropy — Implications for the Second Law of Thermodynamics,” Physical Review A 39 (1989): 5378–5386
“Computational capacity of the universe,” quant-ph/0110141
- Michel Loève, Probability Theory, Princeton, D. Van Nostrand, 1963
- E. Lutwak, D. Yang, G. Zhang, “Cramer-Rao and Moment-Entropy Inequalities for Renyi Entropy and Generalized Fisher Information”, IEEE Transactions on Information Theory 51 (2005): 473–478
- Christian K. Machens, “Adaptive sampling by information maximization,” physics/0112070
- David J. C. MacKay
Information Theory, Inference and Learning Algorithms [>>>]
“Rate of Information Acquisition by a Species subjected to Natural Selection” [>>>]
- Donald Mackay, Information, Mechanism and Meaning [a notion of “meaning” out of information theory?]
- Andrew J. Majda, Rafail V. Abramov, Marcus J. Grote, Information Theory and Stochastic for Multiscale Nonlinear Systems [Sounds interesting, to judge from the >>>. PDF (draft?)]
- David Malone, Wayne J. Sullivan, “Guesswork and Entropy”, IEEE Transactions on Information Theory 50 (2004): 525–526
- Eddy Mayer-Wolf, Moshe Zakai, “Some relations between mutual information and estimation error on Wiener space”, math.PR/0610024
- James W. McAllister, “Effective Complexity as a Measure of Information Content”, Philosophy of Science 70 (2003): 302–307
- Robert J. McEliece, The Theory of Information and Coding, Kluwer Academic Publ., 2002
- N. Merhav, M. J. Weinberger, “On Universal Simulation of Information Sources Using Training Data”, IEEE Transactions on Information Theory 50 (2004): 5–20; +Addendum, IEEE Transactions on Information Theory 51 (2005): 3381–3383
- E. Meron, M. Feder, “Finite-Memory Universal Prediction of Individual Sequences”, IEEE Transactions on Information Theory 50 (2004): 1506–1523
- Sanjoy K. Mitter, Nigel J. Newton, “Information and Entropy Flow in the Kalman-Bucy Filter”, Journal of Statistical Physics 118 (2005): 145–176
- Caterina E. Mora, Hans J. Briegel, “Algorithmic Complexity and Entanglement of Quantum States”, Physical Review Letters 95 (2005): 200503 [“We define the algorithmic complexity of a quantum state relative to a given precision parameter, and give upper bounds for various examples of states. We also establish a connection between the entanglement of a quantum state and its algorithmic complexity.”]
- I. J. Myung, Vijay Balasubramanian, M. A. Pitt, “Counting probability distributions: Differential geometry and model selection”, PNAS 97 (2000): 11170–11175
- National Research Council (USA), Probability and Algorithms [on-line]
- Ilya Nemenman, “Information theory, multivariate dependence, and genetic network inference”, q-bio.QM/0406015
- Ilya Nemenman, “Inference of entropies of discrete random variables with unknown cardinalities,” physics/0207009
- Jill North, “Symmetry and Probability”, phil-sci/2978
- John D. Norton, “Eaters of the Lotus: Landauer’s Principle and the Return of Maxwell’s Demon”, phil-sci 1729
- Peter Olofsson, Probability, Statistics, and Stochastic Processes, Wiley-Interscience, 2005
- Liam Paninski, “Asymptotic Theory of Information-Theoretic Experimental Design”, Neural Computation 17 (2005): 1480–1507
- Liam Paninski, “Estimation of Entropy and Mutual Information”, Neural Computation 15 (2003): 1191–1253 [Preprint; code]
- Liam Paninski, “Estimating Entropy on m Bins Given Fewer Than m Samples”, IEEE Transactions on Information Theory 50 (2004): 2200–2203
- Papoulis, Probability, Random Variables and Stochastic Processes, McGraw-Hill Companies, 1991
- Allon Percus, Gabriel Istrate, Cristopher Moore (eds.), Computational Complexity and Statistical Physics [>>>]
- Denes Petz, “Entropy, von Neumann and the von Neumann Entropy,” math-ph/0102013
- C.-E. Pfister, W. G. Sullivan, “Renyi entropy, guesswork moments, and large deviations”, IEEE Transactions on Information Theory 50 (2004): 2794–2800
- John Robinson Pierce, Symbols, Signals and Noise: The Nature and Process of Communication. HarperCollins (paper), 1961
- A. R. Plastino, A. Daffertshofer, “Liouville Dynamics and the Conservation of Classical Information”, Physical Review Letters 93 (2004): 138701
- Jan von Plato, Creating Modern Probability: Its Mathematics, Physics and Philosophy in Historical Perspective. In: Brian Skyrms (ed.) Cambridge Studies in Probability, Induction & Decision Theory, Cambridge UP, 1998
- David Pollard, A User’s Guide to Measure-Theoretic Probability, Cambridge UP, 2001
- Hong Qian, “Relative Entropy: Free Energy Associated with Equilibrium Fluctuations and Nonequilibrium Deviations”, math-ph/0007010 = Physical Review E 63 (2001): 042103
- Ziad Rached, Fady Alajaji, L. Lorne Campbell
“Rényi’s Divergence and Entropy Rates for Finite Alphabet Markov Sources”, IEEE Transactions on Information Theory 47 (2001): 1553–1561
“The Kullback-Leibler Divergence Rate Between Markov Sources”, IEEE Transactions on Information Theory 50 (2004): 917–921
- Yaron Rachlin, Rohit Negi, Pradeep Khosla, “Sensing Capacity for Markov Random Fields”, cs.IT/0508054
- M. Rao, Y. Chen, B. C. Vemuri, F. Wang, “Cumulative Residual Entropy: A New Measure of Information”, IEEE Transactions on Information Theory 50 (2004): 1220–1228
- Juan Ramón Rico-Juan, Jorge Calera-Rubio, Rafael C. Carrasco, “Smoothing and compression with stochastic k-testable tree languages”, Pattern Recognition 38 (2005): 1420–1430
- Sidney Resnick, A Probability Path, Birkhäuser, Boston 1999
- Pál Révész, The Laws of Large Numbers, Aiadémiai JCiadó, Budapest, 1967
- Mohammad Rezaeian, “Hidden Markov Process: A New Representation, Entropy Rate and Estimation Entropy”, cs.IT/0606114
- Jorma Rissanen, Stochastic Complexity in Statistical Inquiry [Applications of coding ideas to statistical problems.]
- E. Rivals, J.-P. Delahae, “Optimal Representation in Average Using Kolmogorov Complexity,” Theoretical Computer Science 200 (1998): 261–287
“Are We Cruising a Hypothesis Space?”, physics/9808009
“The ABC of Model Selection: AIC, BIC, and the New CIC” [PDF preprint]
“Raping the Likelihood Principle” [Abstract: “Information Geometry brings a new level of objectivity to bayesian inference and resolves the paradoxes related to the so called Likelihood Principle.” PDF preprint]
- Reuven Y. Rubinstein, “A Stochastic Minimum Cross-Entropy Method for Combinatorial Optimization and Rare-event Estimation”, Methodology and Computing in Applied Probability 7 (2005): 5–50
- Reuven Y. Rubinstein, Dirk P. Kroese, The Cross-Entropy Method: A Unified Approach to Combinatorial Optimization, Monte-Carlo Simulation, and Machine Learning. Springer, Berlin, 2004
- B. Ya. Ryabko, V. A. Monarev, “Using information theory approach to randomness testing”, Journal of Statistical Planning and Inference 133 (2005); 95–110
- Ines Samengo, “Information loss in an optimal maximum likelihood decoding,” physics/0110074
- Jacek Serafin, “Finitary Codes, a short survey”, math.DS/0608252
- Matthias Scheutz, “When Physical Systems Realize Functions…”, Minds and Machines 9 (1999): 161–196 [“I argue that standard notions of computation together with a ‘state-to-state correspondence view of implementation’ cannot overcome difficulties posed by Putnam’s Realization Theorem and that, therefore, a different approach to implementation is required. The notion ‘realization of a function’, developed out of physical theories, is then introduced as a replacement for the notional pair, ‘computation-implementation’. After gradual refinement, taking practical constraints into account, this notion gives rise to the notion ‘digital system’ which singles out physical systems that could be actually used, and possibly even built.”]
- Thomas Schuermann, Peter Grassberger, “Entropy estimation of symbol sequences,” Chaos 6 (1996): 414–427 = cond-mat/0203436
- R. Schweizer and A. Sklar, Probabilistic Metric Spaces, Nord-Holland, 1983
- Glenn Shafer, Vladimir Vovk, “The Sources of Kolmogorov’s Grundbegriffe”, Statistical Science 21 (2006): 70–98 = math.ST/0606533
- Claude Shannon, Warren Weaver, Mathematical Theory of Communication [first half, Shannon’s paper on “A Mathematical Theory of Communication,” courtesy of Bell Labs, where Shannon worked.]
- Orly Shenker, “Logic and Entropy”, phil-sci 115
- Paul C. Shields, The Ergodic Theory of Discrete Sample Paths [Emphasis on ergodic properties relating to individual sample paths, as well as coding-theoretic arguments. Shield’s page on the book.]
- Tony Short, James Ladyman, Berry Groisman, Stuart Presnell, “The Connection between Logical and Thermodynamical Irreversibility”, phil-sci 2374
- Wojciech Slomczynski, Dynamical Entropy, Markov Operators, and Iterated Function Systems, Wydawnictwo Uniwersytetu Jagiellonskiego, Krakow, 2003.
- Christopher G. Small, D. L. McLeish, Hilbert Space Methods in Probability and Statistical Inference, Wiley-Interscience, 1994
- Steven T. Smith, “Covariance, Subspace, and Intrinsic Cramer-Rao Bounds”, IEEE Transactions on Signal Processing 53 (2005): 1610–1630
- Ray Solomonoff [publications page]
A formal theory of inductive inference: Parts 1 and 2. Information and Control, 7:1–22 and 224–254, 1964
“The Application of Algorithmic Probability to Problems in Artificial Intelligence”, in Kanal and Lemmer (Eds.), Uncertainty in Artificial Intelligence,, Elsevier Science Publishers B.V., pp 473-491, 1986. [pdf]
Complexity-based induction systems: Comparisons and convergence theorems. IEEE Transactions on Information Theory, IT-24:422-432, 1987.
- Alexander Stotland, Andrei A. Pomeransky, Eitan Bachmat, Doron Cohen, “The information entropy of quantum mechanical states”, quant-ph/0401021
- R. F. Streater, “Quantum Orlicz spaces in information geometry”, math-ph/0407046
- Michael Strevens, Bigger than Chaos: Understanding Complexity through Probability, Harvard UP, 2003
- Daniel W. Stroock, Probability Theory: An Analytic View, Cambridge UP, 2008
- Rajesh Sundaresan, “Guessing under source uncertainty”, cs.IT/0603064
- Joe Suzuki, “On Strong Consistency of Model Selection in Classification”, IEEE Transactions on Information Theory 52 (2006): 4767–4774
- H. Takashashi, “Redundancy of Universal Coding, Kolmogorov Complexity, and Hausdorff Dimension”, IEEE Transactions on Information Theory 50 (2004): 2727–2736
- Inder Jeet Taneja
Generalized Information Measures and Their Applications [on-line]
“Inequalities Among Symmetric divergence Measures and Their Refinement”, math.ST/0501303
- Aram J. Thomasian, The Structure of Probability Theory, MacGraw-Hill, 1964
- Eric E. Thomson, William B. Kristan, “Quantifying Stimulus Discriminability: A Comparison of Information Theory and Ideal Observer Analysis”, Neural Computation 17 (2005): 741–778 [warning against a too-common abuse of information theory.]
- C. G. Timpson, “On the Supposed Conceptual Inadequacy of the Shannon Information,” quant-ph/0112178
- Pierre Tisseur, “A bilateral version of the Shannon-McMillan-Breiman Theorem”, math.DS/0312125
- Hugo Touchette, Seth Lloyd, “Information-Theoretic Approach to the Study of Control Systems,” physics/0104007 [Rediscovery and generalization of Ashby’s “law of requisite variety”]
- Marc Toussaint, “Notes on information geometry and evolutionary processes”, nlin.AO/0408040
- Jerzy Tyszkiewicz, Arthur Ramer, Achim Hoffmann, “The Temporal Calculus of Conditional Objects and Conditional Events,” cs.AI/0110003
- Jerzy Tyszkiewicz, Achim Hoffmann, Arthur Ramer, “Embedding Conditional Event Algebras into Temporal Calculus of Conditionals,” cs.AI/0110004
- Marc M. Van Hulle, “Edgeworth Approximation of Multivariate Differential Entropy”, Neural Computation 17 (2005): 1903–1910
- Nikolai Vereshchagin, Paul Vitanyi, “Kolmogorov’s Structure Functions with an Application to the Foundations of Model Selection,” cs.CC/0204037
- Paul M. B. Vitanyi
“Meaningful Information,” cs.CC/0111053
“Quantum Kolmogorov Complexity Based on Classical Descriptions,” quant-ph/0102108
“Randomness,” math.PR/0110086
- P. A. Varotsos, N. V. Sarlis, E. S. Skordas, M. S. Lazaridou
“Entropy in the natural time-domain”, physics/0501117 = Physical Review E 70 (2004): 011106
“Natural entropy fluctuations discriminate similar looking electric signals emitted from systems of different dynamics”, physics/0501118 = Physical Review E 71 (2005)
- Bin Wang, “Minimum Entropy Approach to Word Segmentation Problems,” physics/0008232
- Q. Wang, S. R. Kulkarni, S. Verdu, “Divergence Estimation of Continuous Distributions Based on Data-Dependent Partitions”, IEEE Transactions on Information Theory 51 (2005): 3064–3074
- Watanabe, Knowing and Guessing, Wiley, N.Y.C., 1969
- Edward D. Weinberger
“A Theory of Pragmatic Information and Its Application to the Quasispecies Model of Biological Evolution,” nlin.AO/0105030
“A Generalization of the Shannon-McMillan-Breiman Theorem and the Kelly Criterion Leading to a Definition of Pragmatic Information”, arxiv:0903.2243
- Benjamin Weiss, Single Orbit Dynamics (CBMS Regional Conference Series in Mathematics) American Mathematical Society, 2000 [Procedures for non-parametrically estimating entropy of suitably ergodic sources, using just one realization of the process.]
- T. Weissman, N. Merhav, “On Causal Source Codes With Side Information”, IEEE Transactions on Information Theory 51 (2005): 4003–4013
- Michael M. Wolf, Frank Verstraete, Matthew B. Hastings, J. Ignacio Cirac, “Area Laws in Quantum Systems: Mutual Information and Correlations”, Physical Review Letters 100 (2008): 070502
- David H. Wolpert, “Information Theory – The Bridge Connecting Bounded Rational Game Theory and Statistical Physics”, cond-mat/0402508
- David Wolpert, “On the Computational Capabilities of Physical Systems,” physics/0005058 (pt. I, “The Impossibility of Infallible Computation”) and physics/0005059 (pt. II, “Relationship with Conventional Computer Science”)
- S. Yang, A. Kavcic, S. Tatikonda, “Feedback Capacity of Finite-State Machine Channels”, IEEE Transactions on Information Theory 51 (2005): 799–810
- Jiming Yu, Sergio Verdu, “Schemes for Bidirectional Modeling of Discrete Stationary Sources”, IEEE Transactions on Information Theory 52 (2006): 4789–4807
- Paolo Zanardi, Paolo Giorda, Marco Cozzini, “Information-Theoretic Differential Geometry of Quantum Phase Transitions”, Physical Review Letters 99 (2007): 100603
- W. H. Zurek (ed.), Complexity, Entropy, and the Physics of Information, Redwood City, 1990
- A. K. Zvonkin, L. A. Levin. The complexity of finite objects and the development of the concepts of information and randomness by means of the theory of algorithms. Russian Mathematical Surveys, 25(6):83–124, 1970